ALL >> Business >> View Article
Artificial Intelligence Training: A Comprehensive Overview
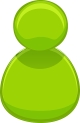
Key Points
AI training involves teaching models to learn from data to perform tasks like prediction or decision-making.
Common methods include supervised, unsupervised, semi-supervised, and reinforcement learning.
Advanced techniques like deep learning and transfer learning enhance model capabilities.
Challenges include data quality, computational demands, and ethical concerns like bias.
Recent advancements (2025) focus on multimodal models and efficient small language models.
Tools like TensorFlow and PyTorch are essential for implementing AI training.
What is AI Training?
AI training is the process of teaching artificial intelligence models to perform specific tasks by exposing them to data. Models learn patterns, relationships, or behaviors from this data, enabling them to make predictions, classify information, or take actions. For example, a model trained on images can learn to identify objects, ...
... while one trained on text can generate human-like responses. The process is foundational to AI applications in healthcare, finance, education, and more.
Core Training Methods
AI training methods vary based on the type of data and task. Supervised learning uses labeled data to teach models input-output mappings, such as predicting house prices. Unsupervised learning finds patterns in unlabeled data, like grouping similar customers. Semi-supervised learning combines both approaches when labeled data is limited. Reinforcement learning trains models through trial and error, rewarding successful actions, as seen in game-playing AI like AlphaGo.
Tools and Challenges
Popular tools like TensorFlow, PyTorch, and scikit-learn simplify AI training by providing libraries for building and testing models. However, challenges persist. High-quality data is crucial, but biases or errors can lead to flawed models. Training complex models requires significant computational resources, and ethical issues, such as ensuring fairness and transparency, are increasingly important.
Recent and Future Trends
As of 2025, AI training is advancing with multimodal models that process text, images, and videos, and smaller, efficient models for specialized tasks. Looking ahead, trends like explainable AI and quantum machine learning promise to enhance transparency and efficiency. These developments suggest AI training will continue to evolve, addressing current limitations and expanding applications.
Artificial Intelligence Training: Methods, Tools, Challenges, and Future Directions
Introduction
Artificial intelligence (AI) has transformed industries, from healthcare to entertainment, by enabling machines to perform tasks that mimic human intelligence. Central to this transformation is AI training, the process of teaching models to learn from data to make predictions, classify information, or generate content. This article provides a detailed exploration of AI training, covering its core methods, advanced techniques, essential tools, challenges, recent advancements as of 2025, and future trends. By understanding these elements, we can appreciate the complexity and potential of AI and its role in shaping the future.
Types of AI Training
AI training methods are categorized based on how models learn from data. Each method suits different tasks and data types, forming the foundation of most AI systems.
Supervised Learning
Supervised learning involves training a model on a labeled dataset, where each input is paired with a correct output. The model learns to map inputs to outputs, enabling it to predict outcomes for new data. For example, a model trained on labeled images can classify whether an image contains a cat or dog. Common techniques include:
Linear Regression: Predicts numerical values, like house prices.
Logistic Regression: Classifies binary outcomes, like spam detection.
Decision Trees: Makes decisions by splitting data into branches.
Support Vector Machines (SVM): Finds optimal boundaries for classification.
Neural Networks: Models complex patterns using interconnected nodes.
Supervised learning is widely used in applications like medical diagnosis and fraud detection, where clear input-output relationships are available (Supervised Learning).
Unsupervised Learning
Unsupervised learning trains models on unlabeled data, tasking them with finding hidden patterns or structures. This method is ideal for exploratory analysis. For instance, a retailer might use it to group customers by purchasing behavior. Key techniques include:
K-means Clustering: Groups data into clusters based on similarity.
Hierarchical Clustering: Builds a tree of clusters for nested groupings.
Apriori Algorithm: Identifies frequent itemsets in transaction data.
Unsupervised learning is valuable in market segmentation and anomaly detection (Unsupervised Learning).
Semi-Supervised Learning
Semi-supervised learning combines labeled and unlabeled data, leveraging the abundance of unlabeled data to improve model performance when labeled data is scarce. Techniques include:
Self-training: The model labels unlabeled data iteratively.
Co-training: Multiple models share predictions to enhance accuracy.
This approach is useful in scenarios like speech recognition, where labeling audio data is costly (Semi-Supervised Learning).
Reinforcement Learning
Reinforcement learning (RL) trains an agent to make decisions by interacting with an environment, receiving rewards or penalties for actions. The goal is to maximize cumulative rewards. For example, DeepMind’s AlphaGo used RL to master the game of Go. Techniques include:
Q-learning: Estimates the value of actions in different states.
Deep Q-Networks (DQN): Combines Q-learning with deep neural networks.
RL is critical for robotics and autonomous systems (Reinforcement Learning).
Advanced AI Training Methods
Beyond foundational methods, advanced techniques address complex tasks and improve efficiency.
Deep Learning
Deep learning uses neural networks with multiple layers to learn from raw data, automatically extracting features. It excels in tasks like image recognition and natural language processing. Key architectures include:
Convolutional Neural Networks (CNN): Process images for tasks like object detection.
Recurrent Neural Networks (RNN): Handle sequential data, such as time series.
Generative Adversarial Networks (GAN): Generate new data, like realistic images.
Transformers: Power advanced language models like GPT and BERT.
Deep learning drives many state-of-the-art AI applications (Deep Learning Overview).
Transfer Learning
Transfer learning uses knowledge from one task to improve performance on a related task, reducing training time and data needs. For example, a model pre-trained on ImageNet can be fine-tuned for medical imaging. This method is widely used in computer vision and natural language processing (Transfer Learning).
Federated Learning
Federated learning enables collaborative training across multiple devices or organizations without sharing raw data, preserving privacy. Local models are trained, and only updates are shared to improve a global model. It’s used in healthcare for patient data analysis and in mobile devices for personalized AI (Federated Learning).
Additional Advanced Techniques
Other notable methods include:
Self-Supervised Learning: Generates labels from unstructured data, reducing reliance on labeled datasets (Self-Supervised Learning).
Reinforcement Learning with Human Feedback (RLHF): Incorporates human evaluations to refine model outputs, common in generative AI.
Retrieval Augmented Generation (RAG): Enhances models with external data sources for improved accuracy.
Tools and Frameworks for AI Training
AI training relies on robust tools and frameworks that simplify model development and deployment. Below is a table summarizing key tools:
Tool
Description
Primary Use
TensorFlow
Open-source framework by Google for machine learning and deep learning.
Building and deploying models
PyTorch
Flexible framework favored for research due to dynamic computation graphs.
Prototyping and research
scikit-learn
Python library for traditional machine learning tasks like classification.
Data mining and analysis
Keras
High-level API for building neural networks, runs on TensorFlow or PyTorch.
Simplifying model development
Hugging Face
Library for state-of-the-art natural language processing models.
NLP tasks and transformer models
OpenAI Gym
Toolkit for developing and testing reinforcement learning algorithms.
RL research and experimentation
These tools enable researchers and developers to implement complex training methods efficiently (TensorFlow, PyTorch).
Applications of AI Training
AI training methods are applied across various domains, as shown in the following table:
Domain
Training Method
Application
Education
Supervised Learning (ANN)
Predicting student performance
Healthcare
Deep Learning (CNN)
Medical image analysis
Retail
Unsupervised Learning
Customer segmentation
Gaming
Reinforcement Learning
Autonomous game-playing agents
Finance
Transfer Learning
Fraud detection with pre-trained models
Privacy-Sensitive Areas
Federated Learning
Collaborative model training without data sharing
For example, in higher education, machine learning models predict student drop-out rates with high accuracy (81.19% using ANN) (AI in Education).
Challenges in AI Training
AI training faces several challenges that impact model performance and adoption:
Overfitting and Underfitting: Models may memorize training data (overfitting) or fail to learn patterns (underfitting), reducing generalization.
Data Quality and Quantity: High-quality, unbiased data is essential, but collecting large datasets is costly, and biases can lead to unfair outcomes.
Computational Resources: Training deep learning models requires significant computational power, often involving GPUs or TPUs, which can be expensive.
Ethical Considerations: Bias in data, lack of transparency, and fairness issues raise ethical concerns. For instance, biased training data can perpetuate discrimination in AI decisions.
Addressing these challenges requires careful data curation, robust algorithms, and ethical guidelines (AI Ethics).
Recent Advancements in AI Training (2025)
As of 2025, AI training is advancing rapidly, driven by new techniques and applications. Key developments include:
Multimodal Models: Models like OpenAI’s GPT-4V and Google’s Gemini process text, images, and videos, enabling tasks like text-to-video generation (AI Trends).
AI Agents: Autonomous agents with advanced reasoning capabilities solve complex problems, resembling human decision-making (Microsoft AI Trends).
Small Language Models: Efficient models, like Microsoft’s Phi, leverage high-quality data curation for specialized tasks, reducing resource demands.
Defense and Security: AI training supports military applications, such as autonomous drones and surveillance systems (MIT Technology Review).
Generative AI: Advances in generative models enable realistic content creation, from text to videos, with applications in entertainment and education (Coursera AI Trends).
These advancements highlight the shift toward versatile, efficient, and application-specific AI training methods.
Future Trends in AI Training
The future of AI training is poised for significant evolution, driven by emerging technologies and societal needs:
Explainable AI (XAI): Models that provide transparent explanations for their decisions will enhance trust and adoption, particularly in critical domains like healthcare.
Automated Machine Learning (AutoML): Tools that automate model development, from data preprocessing to deployment, will democratize AI training.
Quantum Machine Learning: Integrating quantum computing with machine learning could solve complex problems faster, though it remains in early stages.
These trends suggest a future where AI training is more accessible, transparent, and efficient (MIT Sloan AI Trends).
Conclusion
AI training is a dynamic field that underpins the development of intelligent systems. From supervised learning to advanced techniques like deep learning and federated learning, the methods used to train AI models are diverse and continually evolving. Tools like TensorFlow and PyTorch facilitate implementation, while challenges like data quality and ethical concerns require ongoing attention. Recent advancements, such as multimodal models and efficient small language models, demonstrate the field’s rapid progress, and future trends like explainable AI and quantum machine learning promise further innovation. By understanding these elements, we can harness AI’s potential responsibly and effectively, shaping a future where intelligent systems enhance human capabilities across industries.
Add Comment
Business Articles
1. The Evolution Of The Fanny Pack Over The YearsAuthor: iven gayash
2. Powering Sustainable Agriculture With Efficient Pumps
Author: Sundar
3. Step Inside Tomorrow: How Cogito's 3d Walkthroughs Redefine Coimbatore Spaces
Author: cogito
4. How To Pick The Best Civil Contractor In Valasaravakkam For Your Home Project
Author: bharathi
5. Enhancing Your Property With Quality Driveways, Patios, And Pathways: A Comprehensive Guide
Author: Vikram kumar
6. The Importance Of Surface Finishing In Aluminum Products
Author: bqmp
7. Simplifying Global Trade With Workseer’s Advanced Hs Code & Eccn Classification Tools
Author: sumit
8. How To Start A Crab-themed Home Garden For Beginners
Author: bharathi
9. How To Choose The Best Six Sigma Training Institute In Chennai
Author: bharathi
10. Rv Essentials For Long-term Living: What You Really Need
Author: Devon Curran
11. Dubai's Best Laptop Screen Replacement Services: Fast, Dependable Fixes
Author: laptop screen replacement services in dubai
12. Black Magic Specialist In Udupi
Author: astrobest09
13. Steps For Plastic Injection Molding Process
Author: Ryan
14. Understanding Injection Mold Life: Spi Classes And Key Longevity Factors
Author: Ryan
15. How To Identify High-quality Stainless Steel Flanges
Author: Neelam Forge India