ALL >> Education >> View Article
How Do You Apply Pca To Reduce Dimensionality In Datasets?
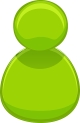
In the realm of data science and machine learning, dimensionality reduction plays a crucial role in improving the efficiency and performance of models. One of the most popular techniques for this purpose is Principal Component Analysis (PCA). PCA allows us to reduce the number of features in our dataset while preserving the most important information. In this blog post, we will explore the steps involved in applying PCA to datasets, discuss its importance, and highlight the benefits of enrolling in a Machine Learning institute to deepen your understanding of this technique.
Understanding Dimensionality Reduction
Dimensionality reduction refers to the process of reducing the number of input variables in a dataset. High-dimensional datasets can lead to issues such as overfitting, increased computational costs, and difficulties in visualization. Techniques like PCA help mitigate these challenges by transforming the data into a lower-dimensional space while maintaining its essential characteristics. By taking a Machine Learning course
...
... with live projects, you can gain hands-on experience in applying PCA effectively.
What is PCA?
PCA is a statistical method that identifies the directions (or principal components) in which the data varies the most. These principal components are linear combinations of the original features and are orthogonal to each other. The first principal component captures the most variance in the data, while the second captures the second most, and so on. By selecting a subset of these components, we can reduce the dataset's dimensionality significantly. If you're interested in learning more about PCA and its applications, consider enrolling in a top Machine Learning institute that offers comprehensive Machine Learning classes.
Steps to Apply PCA
Standardization of Data
The first step in applying PCA is to standardize the dataset. This involves scaling the data so that each feature has a mean of zero and a standard deviation of one. Standardization is essential because PCA is sensitive to the variances of the original features. If one feature has a much larger range than others, it can dominate the principal components. By taking a Machine Learning course with projects, you will learn how to standardize data and understand its impact on model performance.
Covariance Matrix Computation
After standardization, the next step is to compute the covariance matrix of the data. The covariance matrix expresses how much the dimensions (features) vary from the mean with respect to each other. It is a key component in identifying the principal components. Learning how to compute the covariance matrix and interpret its values is an integral part of the curriculum in any reputable Machine Learning certification program.
Eigenvalue and Eigenvector Calculation
Once the covariance matrix is computed, the next step is to calculate the eigenvalues and eigenvectors. Eigenvalues represent the amount of variance carried in each principal component, while eigenvectors indicate the direction of these components. By analyzing the eigenvalues, we can determine how many components to keep. If you are pursuing a Machine Learning course with jobs, you will gain practical experience in performing these calculations using Python or R.
Selecting Principal Components
After obtaining the eigenvalues and eigenvectors, the next step is to rank them and select the top k components that explain the most variance. The choice of k depends on the desired level of dimensionality reduction and the amount of variance you want to retain. A common approach is to look for a cumulative explained variance plot, which helps visualize how many components are necessary to achieve a certain level of variance retention. In a Machine Learning course with live projects, students often work on real datasets to determine the optimal number of components.
Transforming the Data
With the selected principal components, we can now transform the original data. This transformation involves projecting the standardized data onto the new feature space defined by the selected principal components. This step results in a reduced dataset that retains the most critical information while discarding the less important features. Engaging with a Machine Learning coaching program can provide you with the necessary guidance to master this transformation process.
Visualization and Interpretation
Finally, the reduced dataset can be visualized to understand the underlying structure and relationships within the data. Techniques such as scatter plots can be used to visualize the data in the new dimensions. This step is essential for interpreting the results of PCA and understanding how dimensionality reduction has impacted the dataset. Attending classes at the best Machine Learning institute will equip you with the skills to visualize and interpret your findings effectively.
Applying PCA for dimensionality reduction is a vital skill in the field of data science and machine learning. By following the steps outlined above—standardization, covariance matrix computation, eigenvalue and eigenvector calculation, component selection, data transformation, and visualization—you can effectively utilize PCA in your projects. To gain a deeper understanding and practical skills in PCA and other machine learning techniques, consider enrolling in a reputable Machine Learning institute that offers a comprehensive curriculum, including a Machine Learning course with projects and real-world applications. By investing in your education through Machine Learning classes, coaching, and certifications, you can enhance your expertise and open doors to numerous career opportunities in this exciting field.
Add Comment
Education Articles
1. Devops: The Modern Skillset Every Tech Professional Should MasterAuthor: safarisprz01
2. Salesforce Marketing Cloud Training In India | Cloud
Author: Visualpath
3. How An English Medium School Shapes A Child’s Future In Today’s Global World
Author: Mount Litera Zee School
4. Mern Stack Online Training In Ameerpet | Mern Stack Ai Training
Author: Hari
5. Why Online Courses In Sap Sd Are The Best Solution For Today's Professionals
Author: ezylern
6. Sailpoint Online Course In Bangalore For Professionals
Author: Pravin
7. Sap Ai Course | Sap Ai Online Training In Hyderabad
Author: gollakalyan
8. Why Aima Is The Best Choice For A Global Advanced Management Programme
Author: Aima Courses
9. The Best Oracle Integration Cloud Online Training
Author: naveen
10. Mlops Training Course In Chennai | Mlops Training
Author: visualpath
11. International Cbse School In Nallagandla,
Author: Johnwick
12. Best Mba Dual Specialization Combinations For 2025 And Beyond
Author: IIBMS Institute
13. Top Docker Kubernetes Training In Hyderabad | Docker And Kubernetestop Docker Kubernetes Training In Hyderabad | Docker And Kubernetes
Author: krishna
14. Full Stack Web Development Course In Noida
Author: Training Basket
15. Master Advanced Pega Skills With Pega Cssa Infinity'24.2 Online Training By Pegagang
Author: PegaGang