ALL >> Technology,-Gadget-and-Science >> View Article
Future Trends In Data Labeling: Ai-powered Labeling And Beyond
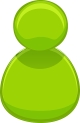
Introduction to Data Labeling
Data labeling is the unsung hero behind the scenes of artificial intelligence, quietly shaping the future of technology. As we dive into the world of data labeling services, traditional methods are making way for innovative AI-powered solutions that are revolutionizing the industry. Join us on this journey to explore the exciting trends and challenges in data labeling, and discover how quality labeled data is crucial for unleashing the full potential of AI.
Traditional Methods of Data Labeling
Traditional methods of data labeling have long been the backbone of training machine learning models. One common approach is manual labeling, where human annotators meticulously tag and categorize data points. This method is time-consuming and prone to errors due to human subjectivity.
Another traditional method involves using in-house teams or outsourcing to third-party services for data annotation tasks. While this can be effective, it also presents challenges such as scalability issues and potential inconsistencies in labeling quality across different projects.
...
... Crowdsourcing platforms have also been utilized for data labeling, leveraging a large pool of contributors to label datasets quickly and at scale. However, ensuring accuracy and reliability can be a concern with this method.
While traditional data labeling methods have laid the foundation for AI development, advancements in technology are now paving the way for more efficient and accurate approaches like AI-powered labeling.
The Rise of AI-Powered Labeling
In the ever-evolving world of data labeling, one trend that stands out is the rise of AI-powered labeling. This innovative approach leverages artificial intelligence algorithms to automate and streamline the data labeling process like never before.
AI-powered labeling systems can analyze vast amounts of unstructured data quickly and accurately, significantly reducing the time and effort required for manual labeling tasks. By harnessing machine learning capabilities, these systems can continuously improve their accuracy over time.
One key advantage of AI-powered labeling is its ability to handle complex datasets with diverse types of information. Whether it's images, text, audio or video data, AI models can efficiently categorize and annotate these different forms of content with high precision.
Despite its many benefits, AI-powered labeling does come with its own set of challenges. Ensuring the quality and reliability of labeled data remains crucial in training accurate machine learning models. Organizations must also address issues related to bias and ethical considerations when implementing automated labeling solutions.
As technology continues to advance rapidly, we can expect further enhancements in AI-powered labeling techniques. With ongoing developments in deep learning algorithms and neural networks, the future holds great promise for more sophisticated and efficient data annotation processes driven by artificial intelligence.
Benefits and Challenges of AI-Powered Labeling
AI-powered labeling offers numerous benefits in the data labeling process. One of the key advantages is its ability to significantly speed up the labeling process, leading to faster deployment of AI models. This efficiency helps organizations save time and resources while improving overall productivity.
Another benefit is the potential for increased accuracy and consistency in labeling tasks. AI algorithms can be trained to maintain high levels of precision, reducing human error and ensuring more reliable datasets for training machine learning models.
Despite these advantages, AI-powered labeling also comes with its own set of challenges. One common issue is the need for large amounts of high-quality labeled data to train AI algorithms effectively. Obtaining such datasets can be costly and time-consuming, especially for niche or specialized domains.
Additionally, there may be concerns about biases in AI algorithms that could impact the quality and fairness of labeled data. It's essential for organizations to implement strategies to mitigate bias and ensure that their AI systems produce accurate and unbiased results.
While AI-powered labeling has its benefits, it's crucial for organizations to address these challenges effectively to harness the full potential of this technology in data annotation processes.
Other Emerging Trends in Data Labeling
As the field of data labeling continues to evolve, other emerging trends are also making their mark. One such trend is the rise of specialized data labeling tools and platforms that cater to specific industries or types of data. These solutions offer more tailored approaches to labeling tasks, ensuring higher accuracy and efficiency in generating labeled datasets.
Another notable trend is the increasing emphasis on privacy and security in data labeling processes. With growing concerns around data protection, companies are implementing stricter protocols and encryption methods to safeguard sensitive information during the labeling process.
Additionally, collaborative labeling models are gaining popularity, where multiple annotators work together on complex datasets to improve annotation consistency and quality. This approach helps address challenges related to subjective labeling tasks by leveraging collective expertise.
Furthermore, we're seeing advancements in active learning techniques that allow AI systems to intelligently select which samples require human input for labeling, optimizing resources and speeding up the overall annotation process. These emerging trends are shaping the future of data labeling services towards greater precision, scalability, and reliability.
The Importance of Quality Data Labeling in Artificial Intelligence
In the realm of artificial intelligence, quality data labeling plays a pivotal role in ensuring the accuracy and reliability of AI algorithms. Data labeling serves as the foundation upon which machine learning models are built, guiding them to make informed decisions based on labeled data. Without accurate labels, AI systems may produce flawed results, leading to potential errors and bias in decision-making processes.
Quality data labeling not only enhances the performance of AI algorithms but also fosters trust among users and stakeholders. By providing clear and consistent labels to training datasets, organizations can improve the overall efficiency and effectiveness of their AI applications. Additionally, high-quality data labeling enables businesses to unlock valuable insights from their data, driving innovation and competitive advantage in today's digital landscape.
In an era where data is hailed as the new currency, investing in quality data labeling services is essential for harnessing the full potential of artificial intelligence technologies. With accurate labels fueling intelligent decision-making capabilities, businesses can leverage AI-driven solutions to drive growth, optimize operations, and deliver superior customer experiences.
In this rapidly evolving digital landscape,
data labeling services play a crucial role in enhancing the accuracy and efficiency of AI systems. Traditional methods are being replaced by more advanced AI-powered labeling techniques, offering benefits such as speed, scalability, and cost-effectiveness. However, challenges like ensuring high-quality annotations and addressing bias must be carefully managed.
As we look towards the future of data labeling, emerging trends such as semi-supervised learning and active learning are poised to further revolutionize the industry. These approaches have the potential to drive innovation and improve model performance significantly.
It is clear that quality data labeling is paramount for the success of artificial intelligence applications. By embracing these future trends and technologies, organizations can unlock new possibilities and stay ahead in an increasingly competitive market. Embracing these changes will not only lead to better AI outcomes but also pave the way for groundbreaking advancements in various industries.
Conclusion
Data labeling services will continue to shape the way we interact with technology, enabling machines to interpret complex information accurately and drive meaningful insights. As we navigate through this exciting era of AI-driven solutions, it is essential for businesses to prioritize robust data annotation strategies that ensure reliable results and foster trust in machine learning algorithms.
The future holds endless possibilities for data labeling services, empowering businesses across all sectors to harness the power of AI effectively. By staying informed about current trends and adopting innovative approaches, organizations can position themselves at the forefront of technological advancement while delivering unparalleled value to their customers.
Add Comment
Technology, Gadget and Science Articles
1. Transforming Talent Acquisition With Gen AiAuthor: Prasanth
2. Top 5 Medicine Delivery Apps Revolutionizing Healthcare: Convenience At Your Doorstep
Author: Simon Harris
3. Virtual Answering Service - The Answer To Success In A Competitive World
Author: Eliza Garran
4. From Chaos To Control: The Future Of Raw Material Inventory Management
Author: Logitrac360
5. Best Ways To Extract Upc, Asin, And Walmart Product Codes
Author: Retail Scrape
6. Crypto Marketing Reloaded: Strategies That Actually Work
Author: AlexeiDj
7. Case Study: Restaurant Menu Data Scraping From Grubhub
Author: Food Data Scrape
8. Austin First: Tesla’s Epic Robotaxi Rollout | Impaakt
Author: Impaakt Magazine
9. How Ai Is Revolutionizing Sales? From Conversation To Conversion
Author: Santhosh Kumar
10. Scraping Zepto, Blinkit, And Jiomart Product Prices For Analysis
Author: Retail Scrape
11. Triax Products In Dubai And The Middle East: The Role Of Official Distributors
Author: blogrme
12. Why Choose Ionic For Your Next App? Expert Insights From Chennai Developers
Author: Trionova Technologies
13. How To Use Magento 2 Language Translation: The Complete Guide
Author: Appjetty
14. Exploring Vetro Power Technology: A Revolutionary Approach To Carpet Protection And Cleaning
Author: Vetro Power
15. Automotive Interiors: Benefits Of Protective Coatings On Fabric & Plastic
Author: Vetro Power