ALL >> Education >> View Article
Choosing Between Statistics And Machine Learning: Determining The Right Approach
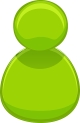
In today's data-driven world, businesses and researchers have a wealth of tools at their disposal, including machine learning, to extract valuable insights from data. Two of the most prominent approaches are statistics and machine learning. While both are indispensable for data analysis, they serve distinct purposes and should be chosen wisely depending on the problem at hand. In this article, we will explore the differences between statistics and machine learning and provide guidance on when to use either one, especially in the context of a machine learning course.
The Foundations: Statistics vs. Machine Learning
Before delving into their applications, let's clarify the foundational differences between statistics and machine learning.
Statistics, as taught in a machine learning course, is a field that has been around for centuries. It is grounded in mathematical theory and focuses on understanding and making inferences from data. Statistics encompasses techniques like hypothesis testing, regression analysis, and analysis of variance. Its primary goal is to uncover patterns, relationships, and insights from ...
... data and draw meaningful conclusions. Statistics often involves making assumptions about the underlying data distribution, such as assuming that data follows a normal distribution.
Machine Learning, on the other hand, as emphasized in machine learning training, is a relatively newer field that has gained immense popularity in recent years. It is a subset of artificial intelligence (AI) and focuses on building algorithms that can learn from data and make predictions or decisions without being explicitly programmed. Machine learning algorithms can be categorized into supervised (predictive), unsupervised (clustering), and reinforcement learning (sequential decision-making). Machine learning is versatile and can handle large volumes of complex data, making it ideal for tasks like image recognition, natural language processing, and recommendation systems.
If you are interested in diving deeper into machine learning, consider enrolling in the best machine learning course or a comprehensive machine learning training program to enhance your knowledge and skills in this exciting field.
When to Choose Statistics
1. Hypothesis Testing: Statistics is the go-to tool for hypothesis testing, often covered in machine learning certification programs. When you want to determine whether a specific intervention had a significant impact, compare groups, or assess the statistical significance of a relationship, statistics provides the necessary tools. For example, in clinical trials, statistical tests are used to determine whether a new drug is effective compared to a placebo.
2. Understanding Relationships:
If you aim to understand the relationships between variables and uncover underlying patterns, statistics, often taught in a machine learning institute, is your friend. Techniques like correlation analysis and regression are powerful tools for exploring these relationships. For instance, statisticians might analyze how temperature affects ice cream sales.
3. Small Sample Sizes: In cases where you have a limited amount of data, statistics can still provide meaningful insights. Statisticians have developed methods to work with small sample sizes, making it invaluable for fields where data collection is expensive or time-consuming.
4. Interpretable Models: Statistics often provides more interpretable models than machine learning. If you need to understand the impact of specific variables on an outcome, linear regression, for instance, can provide coefficients that offer direct insights.
When to Choose Machine Learning
1. Complex Data: Machine learning shines when dealing with complex, high-dimensional data. It excels in tasks like image recognition, speech recognition, and natural language processing. For example, it powers virtual assistants like Siri and Alexa, which understand and respond to spoken language. If you want to enhance your expertise in this field, you can consider pursuing a machine learning certification.
2. Predictive Modeling: If your primary goal is to make predictions, especially in cases where you don't have a clear theory or hypothesis, machine learning, often covered in a machine learning training course, is the way to go. Algorithms like random forests and deep neural networks can capture intricate patterns in the data and provide accurate predictions.
3. Automation: Machine learning allows for automation of decision-making processes. It is commonly used in recommendation systems, fraud detection, and autonomous vehicles, where the system continuously learns and adapts to new data. To become proficient in the field of automation through machine learning, consider joining a dedicated machine learning training institutemachine learning training institute to gain hands-on experience.
4. Large Datasets: When dealing with massive datasets that would be impractical to analyze manually, machine learning offers scalable solutions. Big data analytics, including tools like Hadoop and Spark, often rely on machine learning techniques.
The Hybrid Approach
In many real-world scenarios, a hybrid approach that combines statistics and machine learning can be the most effective. For example, in healthcare, statistical analysis might be used to understand the relationships between patient demographics and disease outcomes, while machine learning models could predict patient risk based on a broader range of factors, including genetic data.
Summary
In the realm of data analysis, the choice between statistics and machine learning depends on the problem you aim to solve. Statistics provides a solid foundation for hypothesis testing and understanding relationships, especially when dealing with smaller datasets. On the other hand, machine learning excels in predictive modeling, handling complex data, and automating decision-making processes, making it indispensable in the age of big data. Ultimately, the best approach often involves a combination of both statistics and machine learning, leveraging the strengths of each to extract valuable insights from the vast sea of data in our digital world. So, when faced with a data-driven challenge, choose wisely and let the nature of the problem guide your decision. If you're interested in delving deeper into either of these fields, you may consider enrolling in a machine learning engineer course or a machine learning training course to gain the necessary skills and knowledge.
Add Comment
Education Articles
1. Devops: The Modern Skillset Every Tech Professional Should MasterAuthor: safarisprz01
2. Salesforce Marketing Cloud Training In India | Cloud
Author: Visualpath
3. How An English Medium School Shapes A Child’s Future In Today’s Global World
Author: Mount Litera Zee School
4. Mern Stack Online Training In Ameerpet | Mern Stack Ai Training
Author: Hari
5. Why Online Courses In Sap Sd Are The Best Solution For Today's Professionals
Author: ezylern
6. Sailpoint Online Course In Bangalore For Professionals
Author: Pravin
7. Sap Ai Course | Sap Ai Online Training In Hyderabad
Author: gollakalyan
8. Why Aima Is The Best Choice For A Global Advanced Management Programme
Author: Aima Courses
9. The Best Oracle Integration Cloud Online Training
Author: naveen
10. Mlops Training Course In Chennai | Mlops Training
Author: visualpath
11. International Cbse School In Nallagandla,
Author: Johnwick
12. Best Mba Dual Specialization Combinations For 2025 And Beyond
Author: IIBMS Institute
13. Top Docker Kubernetes Training In Hyderabad | Docker And Kubernetestop Docker Kubernetes Training In Hyderabad | Docker And Kubernetes
Author: krishna
14. Full Stack Web Development Course In Noida
Author: Training Basket
15. Master Advanced Pega Skills With Pega Cssa Infinity'24.2 Online Training By Pegagang
Author: PegaGang