ALL >> Technology,-Gadget-and-Science >> View Article
How High Performance Computing (hpc) Can Be Used For Ai
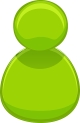
How HPC Aids in the Development of Better AI Applications
The normal number of nodes in an HPC system is between 16 and 64, and each node has two or more CPUs. Compared to conventional systems, which only have one or two CPUs in a single device, this offers noticeably more processing capability. Nodes in HPC systems also contribute memory and storage resources, which results in significantly more capacity and speed than traditional systems.
Field-programmable gate arrays (FPGAs) and graphics processing units (GPUs) are commonly used in HPC systems to increase computing power. These are processors that have been specifically designed to run deep learning techniques, which are at the core of most AI models, a great deal quicker than conventional CPUs.
HPC systems can advance the development of artificial intelligence in a number of ways
Specialized processors
You can process AI-related algorithms, including those employed by neural network models, more effectively with GPUs.
Speed
Co-processing and parallel processing greatly accelerate computer operations. You can handle ...
... data sets and conduct experiments more quickly as a result.
Amount
You can run longer analysis and analyze huge amounts of data with a lot of storage and memory. By doing this, you can increase the precision of artificial intelligence models.
Performance
You can disperse workloads across available computing technologies and resources by using high speed computing and AI. You may maximize resource usage in this way.
Cost
Access to high speed computing with big data and AI-powered can be more affordably provided through HPC systems. You can use HPC with pay-as-you-go pricing and avoid upfront expenditures if you employ cloud resources.
The Merging of AI and HPC
Advances in HPC go hand in hand with the development of AI. Compared to conventional systems, HPC can assist AI model training more effectively. While this is going on, AI can be used to more effectively queue and process workloads, making the most of HPC systems' resources.
AI and HPC both require high-performance infrastructure in addition to the tasks they carry out. These infrastructure requirements include accelerators, high-speed interconnects, enormous amounts of storage and computational power. Users of both technologies are aware of this overlap, and many are attempting to take use of these parallels and synergies to create stronger tools.
The fact that these technologies are controlled in such disparate ways should be noted. AI applications are commonly hosted in containers and managed with Kubernetes, in contrast to HPC workloads, which are frequently managed with Slurm or PBS Pro. The next significant development for these technologies will undoubtedly be overcoming this disparity and combining tooling.
How HPC is Advancing AI
HPC and AI have a wide range of practical applications, including fraud detection and weather forecasting. Three examples are provided below so you may get a better feel of the advantages this pairing can offer.
Automotive
The concept of autonomous vehicles is at least familiar to the majority of people. These are cars that can adjust their lane positions on their own, park by themselves, or dial 911 when an accident occurs. The existing AI capabilities that now assist drivers in navigating and staying safe are expanded upon by autonomous vehicles.
These vehicles must gather, analyze, and analyze enormous volumes of sensor data every second to achieve this. But first, it's necessary to train the algorithms that these cars employ to anticipate events and choose what to do.
HPC systems can be used for this training. In-vehicle HPC systems can be utilized to run these models and process data in real-time after batch size is determined and models are trained. AI models cannot be run quickly enough to be useful or effective without HPC.
Biomedical
Biomedical research is becoming more and more digital as technology develops. Researchers may now access and evaluate huge amounts of biological data. Technologies ranging from cryo-electron microscopes to genome sequencing produce this data. Many academics are using HPC-supported AI-based analytics to process this data.
Drug research is an example of this in use frequently. The biological models used in this research are extremely precise and can simulate biological processes right down to the subcellular level. Then, using AI, these researchers try to predict how various medication combinations and structures would influence how people behave.
Healthcare
Medical decision-making, clinical studies, and patient records all result in the ongoing generation of data in contemporary hospitals. Advanced tools are needed for the efficient analysis and application of this data to enhance patient care, clinician effectiveness, and hospital management. AI models and HPC systems are two examples.
The development of more dependable diagnostic tools is one use of HPC and AI. For instance, image recognition models are being trained to correctly distinguish between malignant and benign tumors in masses. To do this, scientists use massive volumes of generated data produced by HPC computers to train models. With the use of this information, the diagnostic model is being improved in the hopes that it could one day be used to screen patients beforehand or detect malignancies early in
Conclusion
Development services for AI and HPC go together perfectly. When these two technologies are used to complement one another, AI can advance at the rate necessary to grow smarter, and HPC can advance to the level of intelligence required to deliver superior outcomes. AI receives the data it requires to learn using a specific deep learning model when combined with large data. The AI program processes the data more quickly thanks to HPC. The healthcare, biomedical, and automotive industries can all benefit from these new capabilities.
Add Comment
Technology, Gadget and Science Articles
1. Top 5 Mistakes To Avoid With Apostilled Translations In GlasgowAuthor: glasgowtranslationservices
2. Comparing In-house Vs. Freelance Academic Translation In London: Which Is Better?
Author: londontranslationservice
3. Collaboration Features In Video Platforms
Author: Zyan
4. The Role Of Translation Services In The Entertainment Industry
Author: premiumlinguisticservices
5. Kentico To Umbraco Migration: A Complete Guide For Seamless Transition
Author: Addxp Technologies
6. How To Use Data Analytics To Improve Your Marketing Campaigns: Social Media & Emerging Tech
Author: jatin
7. Busch Group Presents Innovative Vacuum Solutions At Battery Show Europe 2025 In Stuttgart
Author: Busch Vacuum Solutions
8. Myteam11 Data Scraping Helps Decode Player Trends For Winning Predictions
Author: Retail Scrape
9. Banners For Trade Shows: Standing Out In A Crowded Marketplace
Author: printitusa
10. Get Complete Solutions When You Get Your Business Tied To A Virtual Receptionist
Author: Eliza Garran
11. The Fundamentals Of A Successful Website That Gives A Winning Edge To Entrepreneurs
Author: Liam Mackie
12. How Seo Can Make Your Website Friendly For Google Page Ranking?
Author: Liam Mackie
13. The Call Answering Service Is A Must For Every Business Type!
Author: Eliza Garran
14. Choosing The Best Compliance Management Software: A Modern Business Imperative
Author: vishva
15. Web Scraping Food Delivery Platforms For Competitive Intelligence
Author: Food Data Scrape