ALL >> Education >> View Article
What Is Predictive Analytics
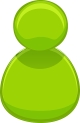
Predictive Analytics
Prediction analytics mixes sophisticated statistics with artificial intelligence to help you predict what will happen next. It’s used in experience management programs to simulate the impact of activities on a company’s bottom line, and it has the potential to change the way businesses make decisions. With our guide, you’ll learn what predictive analytics is, how it works, and how to get started utilizing it.
Predictive analytics defined
Predictive analytics is the use of mathematics, statistics, and data mining to generate predictions about what will happen in the future, using both historical and present data.
The human brain is programmed to make predictions about what will happen next. We use our previous experiences to identify those that are relevant to the current circumstance, and then we use that information to make an educated guess or forecast about future outcomes.
Predictive analytics allows firms to forecast future customer behavior based on historical data and trends. Purchase history, customer service interactions, and demographics ...
... (to mention a few) can all be used to predict customer turnover, prospective future purchases, and lifetime values.
Predictive models are a type of sophisticated “gut” prediction that is objective, repeatable, and data-driven. They organize data and statistics in such a way that it is simple to see trends and make accurate predictions. When you add in the genuine voice of the consumer to detect sentiment, it becomes even more powerful – this includes analyzing data from all feedback channels, including as surveys, social media, service calls, and more.
It goes without saying that the more data you have, the more accurate your forecasts will be. As a result, businesses are increasingly collecting data on their employees, customers, goods, and brands in order to generate more accurate predictions.
That kind of information was hard to come by until lately. Predictive analytics, on the other hand, has become not only more accessible, but also more powerful and advanced than ever before, thanks to the rise of Big Data. We now have the ability to collect massive amounts of data and the processing power to analyze it quickly and efficiently.
What is the distinction between predictive and data analytics?
Predictive analytics is the study of previous and present data to predict future outcomes. Data analytics, on the other hand, is a broader type of analytics that aids businesses in making decisions based on the information they have.
Predictive analytics, on the other hand, is proactive, whereas data analytics is reactive. Both are effective organizational aids, especially when used in tandem.
How does it work?
Here’s a quick rundown of the predictive analytics method and the technology that makes it possible. Feel free to move ahead if you’re less interested in what’s under the hood and more interested in how you might use its power for your business.
Making predictions from data necessitates the development of a mathematical model. This is a technique for determining what you need to know based on previous data, the desired outcome, and the scenario’s known facts.
A predictive model can be thought of as a mathematical representation of reality. It duplicates a real-world scenario or idea and scales it down so that only the bits you’re interested in are included, similar to a scale model or architectural model.
There are some examples of predictive analytics models:
Neural networks: In a manner similar to that of the human brain, it uses previous and current data to anticipate future values.
Classification models: Based on past data, it categorizes information. The majority of the time, it delivers a more comprehensive analysis.
Clustering models: Sorts data into separate groups based on shared characteristics.
Forecast models: Metric value prediction is handled by estimating the values of fresh data using historical data.
Outlier models: Within a data set, it deals with anomalous data entries. It recognizes anomalous data on its own or in connection to other categories and statistics.
Time series model: Focuses on data where the input parameter is time.
To begin building the model, collect all of the data you have on the variables that you believe might be used to predict a specific outcome.
If you’re using machine learning to train a prediction model, you’ll need a “source of ground truth” to compare it to — in other words, historical data. This is essentially a dataset of the desired outcome so that the model can learn from previous events.
When you introduce new data, or the “test data set,” you don’t provide the model your outcome information; instead, you see how well it predicts it based on the “source of ground truth,” using precision and recall metrics or other model accuracy measures, depending on your analytical method.
Assume you’re developing a model to predict whether the sun will shine on a certain day. You’d provide it information on issues like these to train it:
How often it was sunny on the same date in previous years
What the weather was like in the past leading up to sunny days
Any known weather systems that affected sunshine in the past, such as storms or low pressure zones
And so on…
It’s crucial to divide the dataset into training and test sets so that you can analyze how well it predicted a sunny day when you give it your test data set — all the predictors without knowing whether it was sunny or not.
The model would also require precise parameters to specify the desired outcome. You may, for example, describe the number of hours of sunshine and the temperature range that defines a sunny day.
Following the training period, your model should (hopefully) be able to forecast that, for example, bright days are more likely to occur after a rainstorm than they were 50 years ago.
Designing a mathematical model and training it to operate well, even in this simplified example, is a hard and time-consuming task. Perfecting it frequently necessitates the expertise of data specialists. Fortunately, there are solutions that may provide you with all of the power of a mathematical model without the need to create one yourself.
What are the commercial benefits of predictive analytics?
The benefits of predictive technology are theoretically limitless because there are so many different applications. Here are a few common commercial applications for predictive models.
Making preparations ahead of time:
The ability to glimpse into the future and plan accordingly is perhaps the most evident benefit of predictive analytics for business. Predictive technologies can tell you what’s coming up on the horizon so you can plan ahead of time and modify your resource allocation. As an example, imagine you’re a fashion retailer. Natural materials are about to become more popular, according to your predicted model. You can start collaborating with designers and manufacturers who specialize in these types of garments, and reduce your synthetic lines.
Efficient and time-saving:
Predictive technologies can take over a lot of the work involved in low-risk, everyday decision-making, freeing up humans for more useful or high-risk strategic duties. A predictive model, for example, can automate much of the effort involved in calculating a credit score or determining if a simple insurance claim can be paid out. It may require human assistance for outliers or complex cases, but it will relieve staff of a significant amount of work.
Detection of fraud:
A predictive model’s strength is its capacity to discern trends, which means it can detect when something isn’t quite right. Businesses can use predictive technology to spot unusual patterns of activity that could indicate fraud. For example, if a banking customer in the United States begins to make purchases on other continents in a short period of time, the corporation can step in to ensure the account is secure.
Identifying and predicting consumer churn:
Predictive models can learn the patterns of behavior that precede client churn and alert you when they occur. A corporation may be able to keep a customer by acting quickly.
Service to customers:
By learning what customers appreciate and forecasting what they might want next, predictive technology can help organizations give a personalized experience to customers. It can also improve the customer experience in general by providing businesses with a better understanding of normal consumer behaviors and preferences, which they can use to plan and develop experiences.
Analyzing text:
A sort of qualitative experience data is free text (for example, responses written into an open field on a survey or as part of a customer review). Because it varies in form and structure, it is more difficult to process than numbers and rating scales. Text data can be processed at scale using predictive technology, which can discover clusters of words and phrases that indicate specific moods or ideas. It can then generalize them to produce a big-picture analysis that is easy to comprehend.
Predictive analytics in action examples
Banking and finance:
Predictive analytics has been employed by banks for many years. Credit scores rely on data to estimate creditworthiness; for example, the FICO credit score employs statistical analysis to forecast client behavior, including the likelihood of late payments.
Retail:
Predictive analytics is used in retail for personalization, such as analyzing customer behavior across channels, such as online and offline, in order to present highly personalized offers to customers at a granular level. It’s also used for inventory and supply chain management, analyzing supply and demand peaks and lulls to improve product availability.
Marketing:
Marketers may use predictive analytics to qualify and prioritize leads. Marketers can determine the categories that are closest to or furthest from conversion using data on customer behavior. They can, for example, concentrate on prospects that are most likely to act based on previous interactions or those who are on the verge of churning. Marketers can focus more attention on critical relationships and save time and money by using predictive analytics tools.
Utilities and infrastructure:
Engineers and maintenance teams can utilize building monitoring technologies in conjunction with predictive analytics to predict when utilities will fail or need to be replaced. This lowers maintenance expenses while also increasing resource efficiency and availability. Instead of the lights going out unexpectedly, teams can monitor data points across devices and utilities to determine when action is needed.
Healthcare:
Healthcare organizations are improving patient outcomes and detecting and acting on early warning signs of significant medical disorders by linking patient records with other health data. Predictive algorithms can also be used to aid on-site decision-making for individual patients, as well as to inform a larger group of people on what has to be done when and where. Predictive analytics, for example, can assist decide when a patient should return for a checkup based on their medical history — long before any medical concerns arise.
Media and entertainment:
What type of content does the public want to see? Predictive analytics is used by entertainment companies like Netflix and Amazon Prime Video to search current data for hidden insights, such as which genres are well received by specific demographics and when new releases should be released.
The moment has come for predictive analytics.
We’re in the midst of a predictive analytics gold rush right now. The technology is affordable, the knowledge is available, and there is enough historical data to generate truly significant predictions for anything from environmental protection to education and healthcare.
However, while these capabilities are more available than they once were, mastering predictive analytics still provides businesses with a competitive advantage. Although most organizations see the value of predictive technology for themselves and their customers, not everyone is taking use of it — at least not yet.
Words and phrases relevant to Predictive Analytics
If you’re new to predictive analytics, you’re going to encounter a lot of unfamiliar terminology, which can quickly become perplexing. In this section, we define several often used words and phrases in layman’s terms. Some are related to predictive technology, and others may be used interchangeably or synonymously with the phrase predictive analytics.
Machine learning:
Machine learning is a capability that allows technologies to learn and develop their understanding on their own. For example, a streaming service that learns what kind of film you like and recommends similar titles uses this power. Machine learning is divided into two types: supervised (which involves human intervention) and unsupervised (which does not).
I.:
Artificial intelligence (AI) is a term that refers to while machine learning is founded on the idea that robots should be able to learn and adapt via experience, Artificial intelligence (AI) is a broad term that refers to machines that can do tasks “smartly.” Artificial intelligence employs machine learning, deep learning, and other methods to solve real-world problems.
Deep learning
This is a sort of machine learning that is more advanced. Using several, successive layers of processing, a system – commonly an artificial neural net – examines data. Layers interact to create a deep comprehension of complicated ideas, visuals, or processes. For example, deep learning may be used to analyze images and recognize human faces.
Data mining
The process of sifting through massive amounts of data in search of patterns and trends is known as data mining. Although it is an important part of predictive analytics, it is not the only one.
Algorithm
A set of instructions that a computer follows in order to execute a task is known as an algorithm. The algorithm is the foundation of a predictive model in predictive analytics; it becomes a model after being trained on data.
Add Comment
Education Articles
1. Mastering Fire Safety: The Role Of A Fire Safety InstructorAuthor: Qualtec
2. A Global Perspective: The Transformative Impact Of Studying Abroad
Author: Abhilash
3. Exploring Logistics And Supply Chain Management Courses In Trivandrum: Your Path To A Dynamic Career
Author: Capitalits
4. A Student’s Packing List For Germany: Essential Items For Your Adventure
Author: Uniaccess Study Abroad
5. Water Glass
Author: nehashah
6. Choir Lessons In East Bay – Tips To Choose The Best
Author: Pleasanton Academy of Music
7. Top Reasons To Choose The Best Music Studio In East Bay
Author: Pleasanton Academy of Music
8. Azuredevsecopsonlinetraining | Azuredevopstraininginhyderabad,
Author: Renuka
9. Mendix Training In Ameerpet | Mendix Online Training
Author: Teja
10. How Pune's Top Education Consultants Can Help With Scholarships
Author: Aashu
11. Introduction To Azure Databricks? Creation Of Workspace And Managing Workspace
Author: Eshwar
12. What Are Python Developer Roles And Responsibilities?
Author: Sarika
13. The Valuable Function Of Business Analytics
Author: Giri
14. Role Of A Teacher In Early Childhood Education
Author: Kookaburra
15. Debunking Common Data Science Misconceptions
Author: Gour