ALL >> General >> View Article
Real-time Data Streaming Technologies – Complete Guide
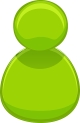
Real-time Data Streaming is data that is created continuously by thousands of data sources, which usually sends data to registers simultaneously, and in small sizes. Real-time data streaming contains a wide range of data such as log records created by customers using your mobile app or web applications, in-game player activity, e-commerce purchases, financial trading floors, information from social networks, or geospatial services, and telemetry from connected devices or instrumentation in data centers. Streaming technologies are at the forefront of the Hadoop ecosystem.
The first point to create when seeing streaming in the data lake is that though many of the offered streaming technologies are very flexible and can be used in many situations, a well-executed data lake offers strict instructions and progressions around ingestion. Data must be ingested, printed to a raw landing area where it can be held, and copied to another area for handling and development.
Kafka is the fresher of the data streaming technologies but is speedily gaining traction as a strong, accessible and fault-tolerant messaging method. Kafka ...
... is more of a transmission, making information “topics” presented to any subscribers who have the approval to listen in. Where Kafka does fall small is in marketable support. Presently, Cloudera contains Kafka, but MapR and Hortonworks do not. Also, Kafka does not contain in-built connectors to other Hadoop products.
Flume has generally been the one choice for flowing ingest and as such, is well-established in the Hadoop ecosystem and is sustained in all marketable Hadoop deliveries. Flume is a push-to-client scheme and works between two endpoints fairly than as a broadcast for any customer to plug into.
Kafka and Flume truly offer connectivity to each other, meaning that they are not necessarily commonly exclusive. Flume contains a sink and a source for Kafka, and there are several documented cases of connecting the two, even in large-scale, production systems.
Once you have a stream of data controlled for your information lake, there are some options for receiving that data into a storable, useable form. With Flume, it’s possible to compose straight to HDFS with in-built sinks. Kafka does not have any in-built connectors.
A storm is a factual real-time handling structure, taking in a stream as a whole “event,” slightly than a sequence of small collections. This means that Storm has very small latency and is well-matched to information that must be consumed as a sole entity. The storm has been used in making instances for the lengthiest of the three results here and has commercial provisions available.
Spark is broadly known for its in-memory treating abilities and the Spark Streaming technologies works on much of a similar basis. Spark is not a truthfully a “real-time” method. Instead, it procedures in micro-batches at distinct breaks. While this presents potential, it also certifies that information is processed constantly, and only once.
Flink is a bit of a hybrid between Spark and Storm. While Spark is a batch structure with no true flowing support and Storm is a flowing structure with no batch provision, Flink contains frameworks for both streaming and group processing. This permits Flink to deal with the small latency of Storm with the information fault tolerance of Spark, besides numerous user-configurable windowing and redundancy settings.
Apache Samza is another spread stream processing structure that is strongly knotted to the Apache Kafka messaging system. Samza is created especially to take benefit from Kafka’s unique style and assurances fault acceptance, buffering and state stores.
We have plenty of choices for processing within a big data system. For stream-only workloads, Storm has wide language provision and so can bring very short latency processing. Kafka and Kinesis are gathering up fast and given that their set of benefits. For batch-only workloads that are not time-sensitive, Hadoop MapReduce is the best choice.
Add Comment
General Articles
1. The Best Education At Barker College: Excellence In Learning And Personal GrowthAuthor: barker
2. What Makes Putty & Slime Toys So Popular In 2025?
Author: La Luna Bella
3. Black Ops 6 Gamescard: What’s Included And Why It’s Worth It
Author: gamescard
4. Your Local Plumbing Experts In Glendale, Ca
Author: Derks Plumbing
5. Effective Turo Rental Data Scraping For Market Analysis
Author: travel
6. Mobile App Development Companies In Florida
Author: DianApps
7. Mern Stack Ai Training | Mern Stack Training In Ameerpet
Author: Hari
8. Discover Paradise: Why Prathamesh Valley Resort Is One Of The Best Resorts In Mahabaleshwar
Author: Prathamesh Valley Resort
9. Unlocking Workplace Productivity With A Sharepoint Intranet
Author: Jessica
10. India’s Role In Supplying nicotine Pouches to Global Markets
Author: Zvol
11. The Complete Guide To Call Center Solutions: Transform Customer Experience In 2025
Author: Anup Jalan
12. Ayurvedic Panchakarna Centre In Rajajinagar
Author: Ayurvedicdoctor
13. Returning To Sports After Partial Knee Replacement
Author: Dr. Amol Kadu
14. Master Math With Abacus Classes In Henderson | Sip Abacus Nz
Author: SIP Abacus
15. Best Cabs In Tirupati For Temple Visits, Tours & Travel
Author: sid