ALL >> Hardware-Software >> View Article
Predictive Analytics: The Power Behind Next-gen Marketing
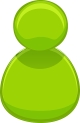
We’ve all had a front-row seat for the marketing transformation: digitization, real-time, social media, content marketing, marketing measurement and dashboards, marketing automation, and inbound marketing. These were not ephemeral ideas. They had the power to transform the very nature and role of marketing–and transform we did during the past decade.
CMOs have been exceptionally adept at identifying and deploying these new ideas throughout their organizations. Just look at some of the new roles and teams our industry has created: Community Manager, Content Marketer, Social Media Manager, Inbound Marketer, Marketing Operations, and Marketing Technologist. They didn’t even exist a few years ago, and today they are central to marketing. Think, too, about how we have brought more accountability and respect to marketing by moving to hard metrics that matter most to the CEO, such as pipeline creation and revenue attribution.
I celebrate the agility of today’s successful CMO. But I also ask the question: What’s next?
Right now, virtually all of our marketing data is backward-looking. ...
... Clicks, Web visits, open rates, downloads, and tweets all happened in the past. What if we could take this data and use it to predict what customers were going to do next? Could we possibly answer questions, such as:
Which of my customers are most likely to terminate service? What steps could I take now to remediate this?
Of the thousands of prospects my sales team are speaking to, which ones are most/least likely to buy? How can I align my sales team’s time with those most likely to buy?
Which of my current customers are most likely to buy some of my other products? Which products should I try to cross-market to which customers?
It is not science-fiction. Right now, the marketing organizations at companies such as ADP, Dell, SunTrust, and Microsoft are doing just that. They are using a variety of statistical techniques to analyze current and historical data to make predictions about the future. It’s called predictive analytics.
An Example: Credit Scores
One of the best-known examples of predictive analytics is credit scoring. The algorithms process a customer’s data, including things like credit history, loan applications, employment, education, and location. The end result is a rank order of individuals by their likelihood of making future credit payments on time. This is your FICO score, and the bank is using the past to make predictions about your future.
Predictive analytics is not some “bright, shiny object” or theory. It is here. Today. Fields as diverse as baseball, insurance, national security, logistics, and (thank you, Nate Silver) presidential elections can now be predicted with stunning accuracy. They answer questions about the future, such as:
Healthcare: Which patients are at risk of developing certain conditions?
Fraud detection: Which transactions are most likely to be fraudulent?
Insurance: What is the risk of illness and death? Of a car accident?
Economics: What is the likely unemployment rate next year?
Online retailing: What book or movie recommendation is most likely to result in an incremental purchase?
Cable television: What offer can I make to this subscriber to prevent her from terminating service?
The key difference between the successful and unsuccessful marketing organizations is how they are scaling up for the data challenge. The amount of information is so vast, and coming in so fast, that humans are no longer able to process it all. Five years ago, it was probably OK to review the data each month (or quarter) for “insights” to incorporate into future marketing programs. For example, you could look at leads coming from trade shows and see which ones actually resulted in closed business, and then calculate the ROI from the event.
Today, however, marketing organizations are scaling these processes by developing predictive models that identify patterns in historical and transactional data. Instead of having marketers crawl over the data in the hope of finding patterns, they are building analytical engines to power through the data.
Think about the recommendation engines of Amazon, Netflix, and Pandora. Humans are not looking at what movies you watched or what books you read. Employees of these companies have built bots to crawl the data, find patterns, and predict what you will want to buy next.
Why Now?
Predictive analytics is gaining traction for three main reasons:
The era of big data is here: Simply put, until recently we didn’t have enough marketing data to confidently predict the future. The amount of data the world produces every two days is equal to all the data produced from the beginning of civilization up to 2003. Today, companies and individuals are spewing out massive amounts of information in social networks, on the Web, and in internal systems (such as CRM and purchase histories). The sheer volume presents an unprecedented opportunity for businesses to gain insights on current and future buying behavior.
Technology is effectively harnessing big data: Advances in technology now allow us to cost-effectively capture, store, search, share, analyze, and visualize data. There have been giant technological advances in computer hardware–faster CPUs, cheaper memory, and massively parallel processing (MPP) architectures. New technologies (Hadoop, MapReduce, and text analytics) can process both structured and unstructured big data. Today, exploring big data and using predictive analytics is within reach of more organizations than ever before.
The democratization of the math: Until recently, big data and predictive analytics were almost exclusively the domain of highly skilled data scientists. Today, software makes even the most exotic of techniques within sight–from simple linear and multivariate regression to classification and regression trees (CART), conditional mutual information algorithms, random forests, and neural networks. While the range of statistical techniques had widened, the availability of graduate students and software has made it more accessible to more organizations. You do not need a small army of PhDs, but you will need to have some familiarity with these methods.
The next generation of marketing leaders will be those who effectively harness the power inherent in big data, and the early adapters are already embracing predictive analytics. If you were an early adapter of marketing automation, then I predict that you’ll also be an early adapter of predictive analytics.
Add Comment
Hardware/Software Articles
1. What Is A Courier Management System In Odoo? A Complete OverviewAuthor: Mayur Meheshwari
2. How Ai Brings Stability To Algorithmic Stablecoin Development
Author: John Parker
3. Destination-style Wedding Venues In Delhi Ncr Without Leaving The City
Author: venueindelhi
4. Testing Skills Every Quality Assurance Engineer And Developer Should Master
Author: brainbell10
5. Marketing Cloud Integration Made Easy With Usa-based Salesforce Developers
Author: Vihan Richard
6. Is Gcc High Mandatory In 2025? Here’s What Federal Contractors Must Know
Author: ECF Data
7. How Online Monitoring Systems Enhance Efficiency In Spinning Operations
Author: Datalog
8. Auto Garage Software In Uae – Boost Your Workshop Efficiency
Author: Auto Garage Software - Masstechme
9. Why A Contemporary Purchase Order Platform Is Necessary To Remove Manual Errors
Author: vishva
10. Best Supplier Management Software For Fast-growing Businesses: A 2025 Review
Author: vishva
11. Ai-powered Workflow Automation For Healthcare Efficiency
Author: Prasanth
12. How Enterprises Are Leveraging Ai In Market Research To Move From Guesswork To Precision
Author: Digital Clerx
13. Harnessing Competitor Product Automatch For Smarter Market Strategies
Author: Prodfinity
14. Best Instagram Clone To Launch Your Own Photo And Video Sharing App
Author: Primocys
15. Why Indian Homes Are Switching To Wall-hung Toilets
Author: plumbing bazzar